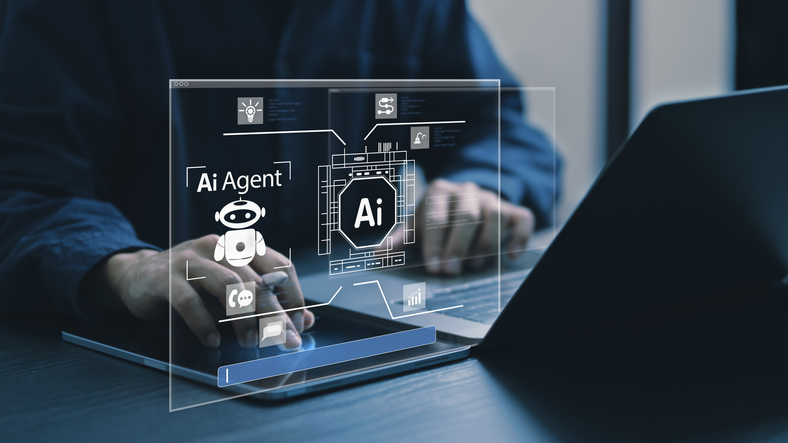
Balancing Innovation with Safety
The rapid evolution of artificial intelligence feels like standing at the edge of a technological cliff. Every leap forward promises ground-breaking potential, but missteps could send us tumbling into chaos. Over the past decade, AI capabilities have surged by an average of 20% annually, reshaping industries from healthcare to logistics. Yet, as these systems grow more sophisticated, so do the risk; unpredictable “hallucinations,” ethical quandaries, and safety gaps threaten to undermine trust.
Consider Tesla’s Autopilot: while its adaptive algorithms enable real-time decision-making, high-profile incidents reveal how even advanced systems can falter without rigorous safeguards. This tension between innovation and reliability lies at the heart of AI’s next chapter.
This article is based on deep research by Alexis from PreEmpt.Life. The full report is avaiable to everyone, free-of-charge. Just click on the link to access.
The Invisible Threats: Hallucinations and Ethical Blind Spots
AI hallucinations. Those baffling, nonsensical outputs aren’t just glitches. They’re symptoms of a deeper challenge: teaching machines to navigate ambiguity. Imagine a medical AI misdiagnosing a patient because it misinterprets an MRI scan, or a financial algorithm triggering a market crash due to flawed data patterns. These aren’t hypotheticals. IBM Watson Health, once hailed as a revolution in oncology, faced criticism when its recommendations sometimes lacked transparency, leaving doctors unsure how to trust its conclusions.
What is the root issue?
Many AI models operate like black boxes. Developers themselves often struggle to trace how decisions are made, especially in neural networks with millions of parameters. Without explainability, errors become mysteries and trust erodes. A 2023 Stanford study found that 68% of businesses hesitate to adopt AI tools without clearer accountability frameworks. The stakes are too high for guesswork.
From Static to Adaptive: The Rise of Self-Learning Systems
Traditional AI models resemble rigid rulebooks. Effective in controlled environments but brittle when faced with chaos. Modern solutions demand flexibility. Take DeepMind’s AlphaFold, which predicts protein structures with astonishing accuracy. Unlike earlier systems, it continuously refines its predictions using real-world feedback, blending biology, ethics, and computational power. This adaptability isn’t just clever engineering; it’s a survival mechanism for AI in dynamic settings.
Real-time adaptive algorithms are becoming the gold standard. These systems analyze live data streams; think traffic patterns for autonomous vehicles or patient vitals in ICU monitoring, to adjust behavior on the fly. Tesla’s Full Self-Driving (FSD) Beta, for instance, learns from millions of miles driven by users worldwide, tweaking its responses to edge cases like sudden pedestrian movements or erratic weather. Yet, this very adaptability introduces new risks: How do we ensure these systems don’t “overfit” to narrow scenarios or inherit biases from flawed training data?
The Human Factor: Ethics, Culture, and the Quest for Transparency
AI’s impact ripples across societies, economies and ecosystems. Consider the European Union’s AI Act, which classifies systems by risk levels and mandates strict oversight for “high-risk” applications like hiring algorithms or facial recognition. Such regulations aim to balance innovation with accountability, but they also highlight a cultural divide: Western frameworks prioritize individual rights, while Asian models often emphasize collective benefit.
Bridging these gaps requires interdisciplinary collaboration. Google’s work on AI ethics teams, comprising philosophers, sociologists and engineers, exemplifies this approach. By integrating diverse perspectives, they’ve developed tools like Model Cards, which document a model’s performance across demographics. Yet, challenges persist. When Microsoft’s Tay chatbot infamously adopted toxic language from Twitter (X) interactions, it underscored the dangers of deploying AI without cultural guardrails.
Case Studies: Lessons from the Frontlines
- Healthcare: IBM Watson’s Pivot
After initial setbacks, IBM retooled Watson Health to focus on explainability. Clinicians now receive “confidence scores” with each diagnosis, detailing how the AI weighed variables like patient history and lab results. This transparency rebuilds trust while empowering doctors to make informed decisions. - Autonomous Vehicles: Waymo’s Safety Net
Waymo’s self-driving cars log billions of simulated miles to test rare scenarios—a child chasing a ball into traffic, a construction zone with conflicting signage. By combining simulations with real-world data, they’ve reduced disengagement (human takeovers) by 75% since 2020. - Climate Science: Microsoft’s Planetary Computer
This AI-driven platform aggregates environmental data from satellites, sensors, and research papers to predict deforestation or track endangered species. It’s a testament to how adaptive systems can tackle global challenges, but only when paired with ethical oversight to prevent misuse.
Building a Safer Tomorrow: Strategies for Decision-Makers
- Invest in Explainability
Tools like Local Interpretable Model-agnostic Explanations (LIME) dissect AI decisions, showing users which factors swayed outcomes. For instance, a loan approval AI might reveal that income level weighed more heavily than credit history. - Embrace Decentralized Learning
Federated learning, used by Apple’s Siri, allows devices to learn collaboratively without sharing raw data. This preserves privacy while improving models—a win-win for industries handling sensitive information. - Cultivate Ethical Agility
Salesforce’s Office of Ethical AI conducts “algorithmic audits” to spot biases in customer-facing tools. Regular audits, paired with employee training, embed ethics into the development lifecycle. - Prepare for the Unpredictable
OpenAI’s “red teaming” exercises stress-test models by simulating adversarial attacks. These drills expose vulnerabilities before deployment, much like cybersecurity penetration testing.
The Road Ahead: Collaboration or Chaos?
The future of AI is a relay race. Success hinges on passing the baton between developers, regulators and end-users. South Korea’s AI Seoul Initiative, launched in 2024, exemplifies this ethos. By uniting government, academia, and tech giants like Samsung, they’ve established sandboxes for testing AI in controlled environments—accelerating innovation while mitigating risks.
Yet, urgency remains. A 2025 Gartner report warns that 45% of organizations lack clear AI governance frameworks, leaving them vulnerable to regulatory blowback or public backlash. The clock is ticking.
Final Thought for Visionaries
As AI reshapes our world, complacency is the enemy. The choices we make today, whether to prioritize transparency over speed, ethics over expediency, will echo for decades. At PreEmpt.Life, we’re committed to guiding leaders through this labyrinth. Our decision intelligence platform combines strategic foresight with real-time horizon-scanning, helping you navigate AI’s complexities with confidence and with ethics and sustainabilty built-in.
Are you ready to future-proof your AI strategy? Explore PreEmpt.Life today, where innovation meets integrity and tomorrow’s challenges become today’s opportunities.